In a DSP’s endless quest to make smarter predictions and better bids, campaigns — yes, even programmatic campaigns — still require some amount of manual work from an account manager. Our own advanced machine learning, with multiple neural networks predicting outcomes in real time, is but one component of what drives ROAS for our advertisers. The other is human intervention. Daily hands-on optimizations are vital if campaigns are to achieve their highest possible performance and this can mean combing through each campaign multiple times a day, adjusting the value of bids, or pausing a poor performing ad in the middle of the night.
The fine tuning process that is campaign optimization can be hours of manual grind, pulling an account manager away from the more strategic and creative work of campaign planning — a process which requires a thorough knowledge of the app ecosystem, a client’s business and their competition. An account manager’s time and expertise should be spent crafting the best possible UA strategy to meet their clients’ objectives, not just pulling the proverbial performance levers. This is why we created CoPilot, our rule-based optimization tool which automates actions that have been tested and proven to further increase ROAS.
Not all DSP’s have identical rule-based functionalities, so it’s important to understand how a DSP defines, executes, and evaluates the success of their rule-based tools and what noticeable advantages can be expected. CoPilot helps advertisers get the most out of their campaign by improving performance in two key areas: model predictions, and value of conversions.
How does CoPilot work?
CoPilot works just like any other set of automated rules. A rule triggers a predefined action when a specific criteria is met. When a campaign runs, CoPilot analyzes several performance metrics that are sliced and diced by different contextual signals, called cells. If a condition is met for the selected criteria within a given time frame, the defined action takes place. The criterion that will trigger an action can be either a positive or negative event and the triggered action can be bidding up, bidding down, or pacing down eligibility of a given subset of supply based on the predefined cell. If conditions are not met, then no changes are made to the campaign.
There is also flexibility with how CoPilot rules can be structured. They can be as simple as a single rule or as complex as multiple rules based on many contextual signals, depending on what your campaign needs to succeed.
An example of a simple pacing rule, where the cell is defined as AppStore ID, would be:

An example of a slightly more complex bid adjustment rule, where target ROAS is 10%, would be:
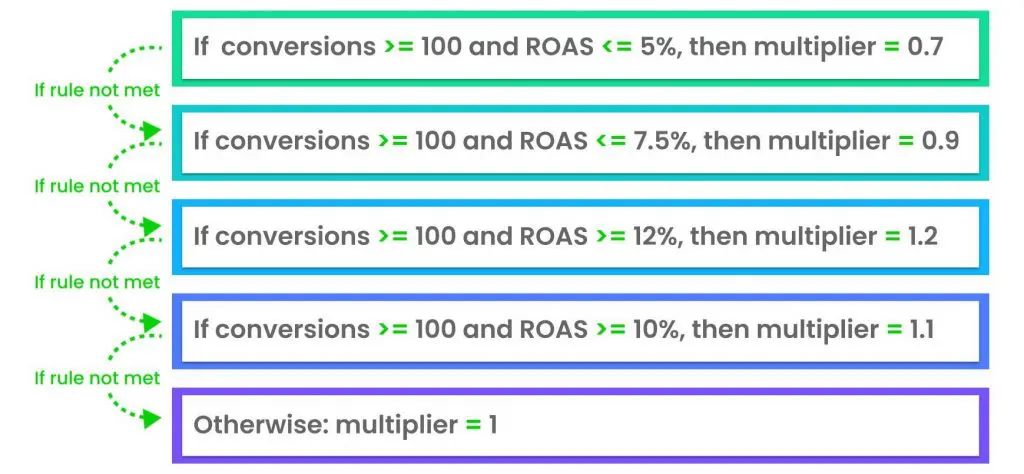
LifeStreet is now ingesting 30+ new contextual signals from its supply partners, all of which can be used to set up fully customizable automated rules. CoPilot can also A/B test up to four rules simultaneously to determine which rule improved the success metric the most.
Safeguard against undesirable results
Automated rules can function as a safety net, monitoring campaigns 24/7. This can help safeguard against unwanted outcomes, such as overspending, and ensures ad dollars are spent only on traffic that converts. Some examples of rules that we have setup using CoPilot include:
- CPI rules: Decrease bids if CPIs are above target.
- Spend rules: Decrease spend on a high spending or new piece of supply until downstream performance is known.
- Payer rate rules: Increase bids if payer rate is above a certain percentage.
- ROAS rules: Pace down a specific piece of supply if D7 ROAS is not hitting goal.
- Retention rules: Bid up pieces of supply where retention is above goal.
For example, if spend on a new app picks up over the weekend, and that new app has a weak conversion rate, instead of waiting until Monday for the account manager to decrease spend on this new piece of supply, the pre-set rule would decrease spend immediately.
Speed and scale
Try as one might, a human simply is unable to ingest and analyze the amount of data at the speed which a machine can. An “if/then” rule can trigger a model to autoscale its media buying based on a target CPI; a decision that could take an account manager hours to calculate and apply across all relevant campaigns. By automating the tedious and time consuming work of regular campaign monitoring and making routine adjustments, CoPilot makes it easier and faster for an account manager to identify what is or isn’t working.
Every ten minutes Copilot reviews, analyzes and adjusts every single campaign running through our platform in seconds–exponentially faster than the hours it would take for a human to do the same. This gives account managers up-to-the-minute macro-level reporting that can be used to monitor the impact and results of CoPilot on their campaigns, while having more time to spend on strategic initiatives.
Automated rules do not mean hands off! Human intervention is still a critical component of campaign performance which includes the creation, testing and implementation of rules-based optimizations. At LifeStreet we like to think of our account manager as the pilot during the flight of a campaign (no pun intended). He or she is the person who has final authority and responsibility for a campaign’s performance. And just like in the aviation world, the copilot is there to assist.