MoPub recently sat down with LifeStreet’s Luke Matkins, VP Technology, to discuss the impacts of iOS 14 on bidding strategies, machine learning’s tie to advertiser performance, how to better track campaign performance, and more. Since 2015, Luke has been responsible for LifeStreet’s machine learning platform, which provides real-time install rate and user quality predictions that drive LifeStreet’s bidding strategies. This month, he’s also been spending a lot of time developing ever more elaborate schemes to effectively use Apple’s 100 distinct campaign IDs.
1. Although Apple recently announced that IDFA changes will be delayed, they’re likely still coming early next year. What are your initial takeaways on the impacts of the iOS 14 IDFA changes when they do happen?
Recently, I happened to be reading “Capital Ideas” by Peter Bernstein and came across a quote from Hayne Leland from back in 1976. At the time, Leland was a finance professor at University of California, Berkeley. Leland worried about his career and financial prospects. After a particularly sleepless night, he decided that “lifestyles were in danger, and it was time for invention.” He went on to create the concept of portfolio insurance, which transformed the financial industry over the next decade.
Portfolio insurance aside, Leland had the right attitude. When the status quo is in danger, it’s time for innovation. With the introduction of iOS 14’s IDFA changes, significant changes will be required across the entirety of the mobile advertising ecosystem. On the demand side, bidding strategies will change as bidders shift towards contextual optimization. On the supply side, new signals will help supplant the loss of device profiles.
The MMPs will figure out new ways to assist advertisers in tracking campaign performance, and advertisers will rethink how campaigns are managed across the board. All of this will create a tremendous amount of churn, but this process of experimentation and adaptation is what will allow us to survive and thrive in the changing world going forward.
2. How will machine learning-backed bidding strategies adapt to further drive advertiser performance?
Without the device ID to link together historical profile data, teams must develop alternate predictive signals.
As one example, at LifeStreet, we’ve created a new type of model to help characterize applications. Especially on the long tail of supply, it can be hard to estimate performance for applications with few training events to learn from. Humans are faced with a similar problem when trying to decide whether to install a new app. They must generalize from their past experience to make predictions about something new.
We realized that application screenshots were a missing piece of the puzzle: we needed to teach our models to “see”. We gathered hundreds of thousands of screenshots and trained a model to recreate these images from the patterns it learned. This allowed the model to identify apps with visual similarities (i.e. apps that have candies, or similar color schemes, etc.) and summarize its learnings into short summary vectors of numbers which describe each image. Afterwards, we provided these summary vectors as additional inputs to our install rate and purchase propensity models so that they could benefit from the image-based generalizations.
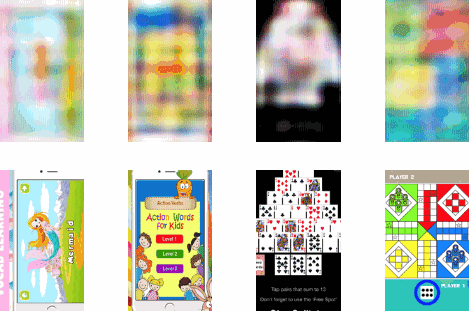
The above example shows the visual application similarity model at work. The bottom row shows ground truth screenshots, and the top row shows the model’s recreated images. Even in this initial version, the model is able to capture a large amount of the information from the summary vectors alone. We are currently using it in production and are seeing improvements in our downstream results. It’s in the early stages, but we’re optimistic that these and other similar developments will continue to drive advertiser performance through iOS14 and beyond.
3. What can exchanges do to help provide additional context around bidding behavior?
Exchanges are in a unique position to help inform bidding behavior. We think there will be a growing diversity of exchange-supplied signals over time, as exchanges continue to differentiate themselves by providing additional information to help bidders make more informed decisions.
Some of the new exchange-supplied signals will provide reliable session depth tracking. Instead of having advertisers estimate session depth themselves by observing bid requests from the device, the exchange SDKs can provide session tracking on the device itself and can supply these signals as part of the bid requests, all without requiring device IDs to be passed along. In addition to the privacy benefits, it’s a more accurate source of this information, as it doesn’t require bidders to have observed every bid request from each device.
Exchanges such as MoPub are also thinking about a variety of other extension fields to the OpenRTB bid request protocol. For instance, these may indicate the bundle and advertiser domain of the previous impression shown to a user in the same slot. Completion parameters may characterize how frequently users view full rewarded or video ads. There are also proposed extensions to indicate battery charging state, low power mode, bluetooth connectivity, disk space usage, and many more device specific properties. In addition, IDFVs may be supplied which will allow bidders to reliably perform their own app sessionization.
Down the road, we think it’s likely that exchanges will continue to create new and useful signals that meaningfully improve predictions. In the long run, it would not surprise me if we transitioned to multiple exchange-specific predictive models that coevolve with the signals available. In this world, performance testing will be critical as teams iterate on their models to find the best drivers of performance.
4. Any opinions on how people will continue to track campaign performance?
Advertisers have historically taken for granted the ability to slice up performance by a myriad of possible dimensions. They can track performance by device type, supply source, advertisement size and format, country, and region, among many others. We at LifeStreet also continuously measure the performance of the various predictive models and strategies that drive our bids.
Having only 100 Apple campaign IDs per product forces a sea of change in performance tracking, and we anticipate a lot of creativity in how exactly we work within this new constraint. We’re playing around with schemes in which we stage multiple mapping strategies over time. For example, we might evaluate a creative test one week and a model test in the following week.
Since Apple’s SKAdNetwork postbacks include source application ID as well as the Apple campaign ID, we’re also envisioning schemes in which campaign mappings can change on a per-application basis. This will allow us to use the 100 campaign IDs most intelligently. For example, if we only buy Words With Friends inventory on MoPub, we don’t need to spend the additional campaign IDs to represent other exchanges. Ideally these mappings will happen dynamically so as to limit the need for manual involvement. It’s going to be a wild ride, and I can confidently predict that we’re all going to spend inordinate amounts of time figuring out how to best use these 100 identifiers.
Article reposted with permission from MoPub.