The global contextual advertising market is set to reach $335.1 billion by 2026 and for good reason. With data privacy restrictions changing advertising on desktop and mobile, advertisers are racing to innovate new ways to serve ads to users that win.
Contextual targeting is nothing new. Demand-side platforms (DSPs) — the technology providers that serve mobile ads to users through the ad exchanges — have had access to contextual signals since mobile advertising began. What’s new are the innovative ways advertisers are using this data to serve hyper-relevant mobile ads that are also privacy compliant. Below, learn how you can use contextual targeting to win more mobile users for your brand or product.
Why is mobile contextual targeting having a renaissance?
Apple began enhancing its data privacy policies long before the deprecation of the IDFA. In 2016, when Apple released iOS 10, it gave users the ability to choose whether or not advertisers received data about their activity through a new limit ad tracking (LAT) feature. While this prompted some advertisers to fine-tune their machine learning (ML) models to scale performance without the IDFA, most advertisers continued to rely on device IDs since only a small percentage of iOS users enabled the LAT feature. Three years after its launch, only 16% of iOS users had enabled LAT. By mid-2020, that rate increased to 25%.
In 2021, when Apple unveiled its ATT Framework, it took its data privacy changes even further. With iOS 14.5 came new restrictions to the collection and use of Apple’s device identifier, the IDFA. Today, unless a user opts in to sharing their IDFA, app marketers do not have access to the behavioral data available through their device ID.
Instead, what marketers do have access to — regardless of whether a user opts in or not — are contextual signals. This data relays useful information about the environment in which a mobile ad appears; such as the app or mobile web URL. Contextual signals also provide broader information about the context of a user’s ad experience; such as their “geo” or location, device type, operating system (OS), their device’s battery level, whether their device is plugged in and charging, and even the text size and brightness their screen is set to. These signals are informative, privacy-compliant, and used by DSPs to make correlations that help them serve relevant ads to iOS users post-IDFA.
While different ad exchanges pass different contextual signals back to DSPs about impression opportunities, certain signals — like those mentioned above — are commonly passed.
What is contextual targeting?
As mentioned above, contextual signals have always been available. There’s just been a renewed focus on contextual data since the restriction of the IDFA and user-level data.
In fact not much else has changed. Today’s bidding formulas use the same prediction models and bidding strategies to target ads to users as they did prior to iOS 14.5. The only difference is the signals being fed into the machine learning (ML) models are privacy-compliant contextual signals instead of behavioral signals.
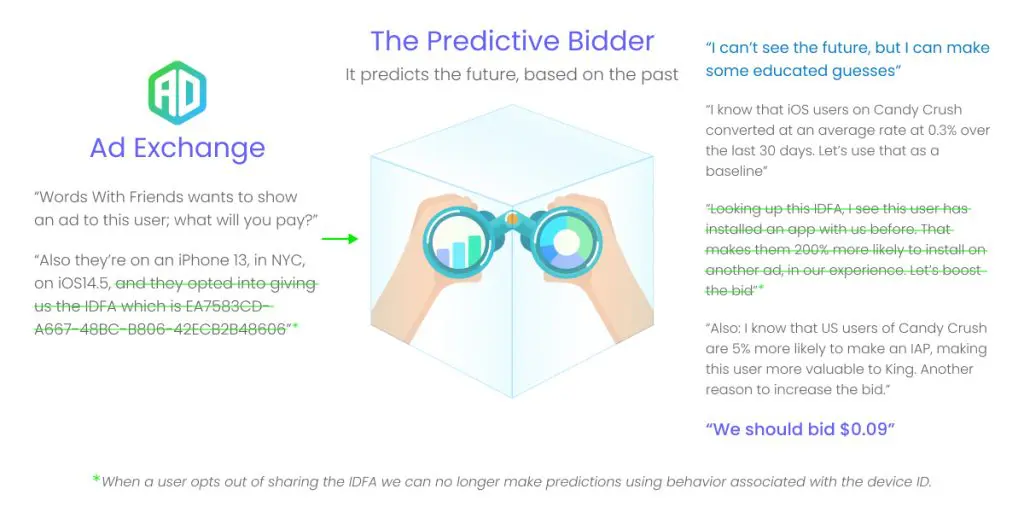
An example of contextual targeting
A gaming app is looking to find audiences that won’t just download an app but also make a first in-app purchase (IAP) within 30 days of download. Every time there is a bid request, the DSP receives a set of contextual signals from the ad exchange about the impression opportunity. These signals include what kind of device and app or mobile URL a user is on who could be served an ad.
In order to bid the right price for the right impression, the DSP’s bidding strategy uses predictive ML models that have been trained on existing users with similar behavior patterns (i.e., an IAP was made within 30 days of an app download). These models look for common features – context clues – between the DSP’s historical impression data and existing users to determine in real time whether or not to bid on an impression and by how much.
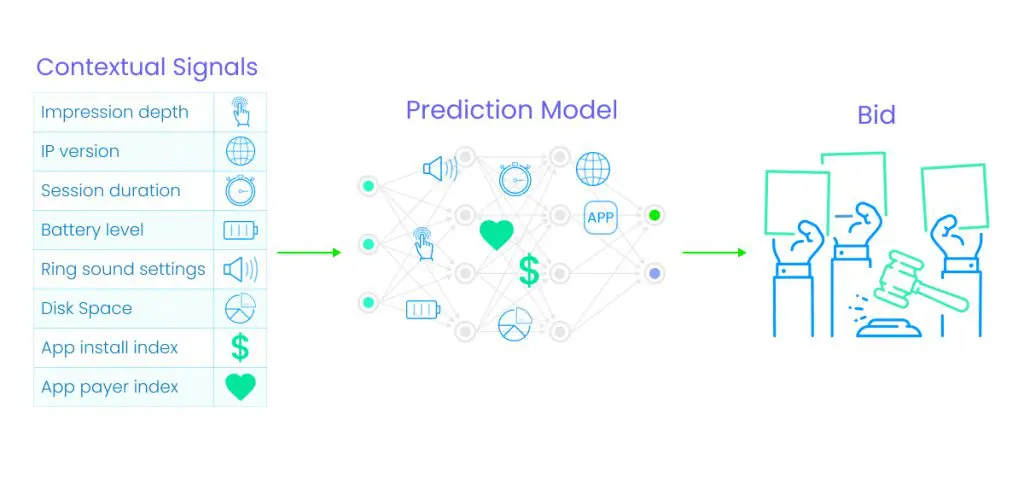
This process is the same whether an app is looking to serve an ad impression in an app that leads the user to download another app; or, whether an app is looking to take a user from a mobile app to a web page to take a certain action, like submit their email or watch a promotional video. Regardless of whether an advertiser has an app or not, in-app contextual targeting can be leveraged by all performance marketers trying to reach their target audience.
How DSPs are innovating contextual targeting
Just as with device ID data pre-ATT, all DSPs have access to the same contextual signals that ad exchanges provide. What separates DSPs are the specific data points they choose to receive and how they use them. Today, ML predictive models focus largely on contextual signals versus behavioral signals to squeeze as much information as possible from an ad impression.
This includes making correlations between contextual signals and what they might indicate about an audience. For example, if an ad impression opportunity in a hyper casual game occurs for a short session in the middle of a weekday, it could be assumed that the user likes to play casual games for short bursts between work. It could also then be assumed that this same user might download a similar hypercasual app if served an ad during a similar midday window.
How we’re innovating contextual targeting at LifeStreet
At LifeStreet, we’ve built a few ways to use contextual information to deliver hyper-relevant campaigns without relying on the IDFA. One is by building bidding models using historical data collected from our extensive experience running LAT campaigns. Even before Apple enforced its Limit Ad Tracking (LAT) framework, we were building predictive models that didn’t use the IDFA but had close to the same predictive accuracy as those reliant on it.
We’ve also built a new feature for our Nero platform called App Mapper. This product ingests large amounts of information about apps from the App Store to make powerful correlations between apps. For example, we can cluster or “map” connections between apps to help improve our bidding predictions even further. This can help our partners boost ROAS by identifying and focusing on better performing apps in which to serve ads.
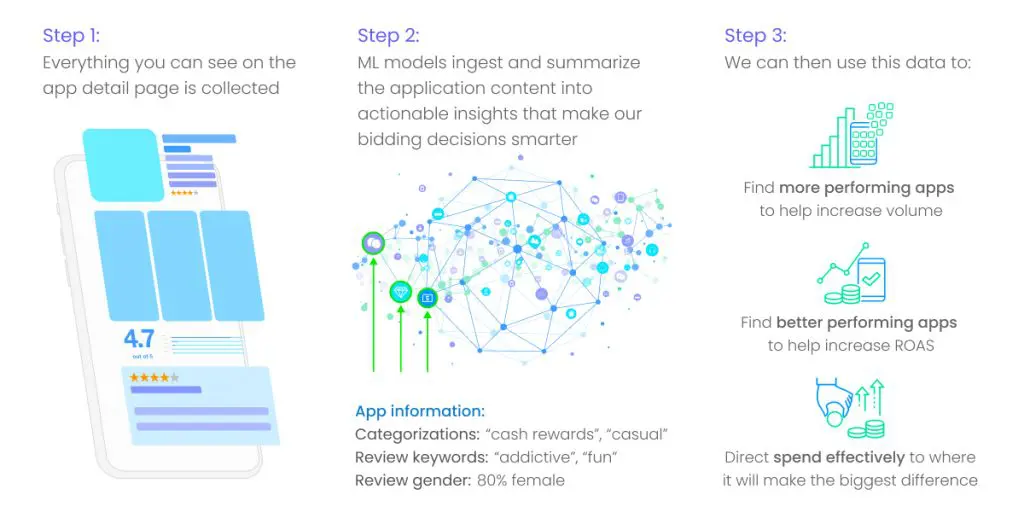
It can also help our partners discover mobile inventory that’s similar to their product’s genre, features or app store details to hit their KPIs. Overall, App Mapper helps our account managers and partners buy more effectively across apps by directing spend to where it will have the greatest impact.
It’s not about the data, but how you use it
Rather than kicking off the mobile advertising apocalypse, the deprecation of the IDFA has simply changed the game. Today, contextual targeting is having a renaissance, but as with most trends in mobile, that could change too. No matter what data is available, DSPs will innovate ways to use it to advertise to in-app audiences. In the new age of data privacy, what will give advertisers an edge will be how their DSP partners use their ML models to make new and unique connections between available data and audiences.